1 Introduction
Over the past two decades, affective scientists and emotion theorists from different traditions have begun to describe emotions as ‘emergent’ properties. The cognitive appraisal theorist Scherer (2009a, 2009b) has characterised emotions as emergent properties of dynamical systems, and Giovanna Colombetti (2014, p. 78) has described emotions as ‘emergent organismic patterns’. The psychological constructionist Lisa Feldman Barrett (2006b, 2009, 2014) has identified emotions with emergent phenomena constructed from more primitive psychological ‘building blocks’, while James A. Coan (2010; 2015) has outlined an ‘emergent variable’ model of emotion that uses statistical methods to define emergentist theories of emotion.
The concept of emergence itself has a long and controversial history, first coming to prominence in the hands of the so-called British emergentists — J. S. Mill, C. D. Broad, and Samuel Alexander — in the late nineteenth and early-twentieth centuries. Following a period of relative neglect during the mid-twentieth century, discussions of emergence have once again become a locus of philosophical attention, motivated in part by increasing deployment of the concept across the sciences. It has been argued that deployment of the concept offers explanatory gains across various scientific disciplines (Mitchell, 2012), while others note that an overly liberal use of the concept threatens trivialising the notion (Sartenaer, 2016).
Stated simply, emergence refers to a part-whole relation such that the whole is both determined by its parts, but has some novel features with respect to them. Though characterised variously in the philosophical literature (e.g., Chalmers, 2008; Guay & Sartenaer, 2016; Humphreys, 2016; Winning & Bechtel, 2019), it is generally agreed that the following two conditions must be met for a higher-level property of some basal-level process or structure to count as an ‘emergent’ (E) product of its base (B). These conditions demand that E be in some sense determined by B (the ‘determination condition’) whilst at the same novel with respect to B (the ‘novelty condition’).1
In the emotion literature, emergence talk appears within the dynamical systems approach to emotion (Coan, 2010; Colombetti, 2014; Lewis, 2005; Lewis & Granic, 2000; Meuleman, 2015; Scherer, 2009a; Walsh, 2021). This approach applies concepts and formal modeling tools from dynamical systems theory (DST) — a branch of mathematics used to describe the behaviour of complex systems that evolve over time — to the explanation of emotional processes. Colombetti (2014, p. 58) notes that dynamical approaches in affective science have ‘an undeniably discursive and speculative character’ and, as yet, only advance ‘quite general hypotheses about [the] emergent character [of emotions]’. Missing from this literature is an explanation of whether and how emotional phenomena meet the two conditions for emergence that form the focal point for discussing emergence in the philosophical literature.
I seek to address this gap here. The article begins by introducing the dynamical approach to emotion (Section 2), and then considers how the two conditions for emergence (determination and novelty) might apply to it (Section 3). I here adopt a ‘pattern emergence’ approach (Humphreys, 2016; Winning & Bechtel, 2019), upon which the emergent phenomenon of interest is a new pattern appearing in the life of a complex system, rather than a new entity or property. I then propose developing the pattern emergence approach to shed light on one of the major conceptual and empirical challenges emotion theorists have faced over the past century: identifying and measuring the presence of emotional episodes (Hollenstein & Lanteigne, 2014; Lindquist et al., 2012). Following Colombetti (2014), I dub this issue the ‘boundary problem’ and discuss it in Section 4. I aim to show that a suitably developed conception of emotions as emergent properties can explain the historical difficulty the field of emotion science has faced in resolving the boundary problem.
To better understand the problem, consider that emotions appear to occur over discrete periods of time, in opposition to, say, moods, or periods of relative emotional neutrality.2 How do we know if an emotion is present or not present? The answer generally accepted in emotion theory is that an emotion is present when it’s components co-occur for some temporal period. These components include appraisal, action tendencies, physiological changes, facial and vocal expression, and subjective feeling (Hutto et al., 2018; Scherer & Moors, 2019). For example, an emotional episode of fear might be characterised as a pattern of coordinated changes comprising a threat appraisal, fearful facial affect, elevated sympathetic arousal, and an urge to flee a threatening situation (Hollenstein & Lanteigne, 2014).
The boundary problem arises because the component processes that comprise an emotional episode are involved in ongoing homeostatic processes that aren’t limited to emotion. Consider fear. Craniofacial muscles produce fearful faces, but are also used for biting, forming sounds, and squinting. Sympathetic nervous system arousal occurs in fear, but is also involved in modulating breathing rate, hormone flow, and intestinal motility. In other words, emotional and non-emotional episodes are made up of the same ‘stuff’. But if the building blocks of emotion just are the building blocks of the human organism, and emotional episodes are like waves in a sea of ongoing physiological and psychological fluctuations, how do we tell the two apart?
A possible solution is to differentiate at least some types of emotion (e.g., anger, fear)3 from ongoing homeostatic processes on the basis of distinctive patterns or ‘signatures’4 formed for the duration of the episode. Various criteria have been offered to identify such patterns (Loaiza, 2021; Scarantino, 2015), and how we conceptualise these criteria matters when assessing the relevant bodies of empirical data. The data have been interpreted variously: as providing some support for the existence of emotional signatures, weak support, or no support at all (Barrett, 2006a; Hollenstein & Lanteigne, 2014; Lench et al., 2011; Lindquist et al., 2012; Scarantino, 2015). This is because whether or not the evidence supports the existence of emotional signatures depends on how a pattern or signature is itself defined (Loaiza, 2021).
Having outlined the boundary problem, I propose explicating the claim that emotions are emergent properties with reference to the notion of ‘constraints’ (Section 4). The term has its roots in classical physics but has been used more recently to explain mechanisms in biological systems (Bechtel, 2017; Hooker, 2013; Pattee, 1971; Winning & Bechtel, 2018). Constraints limit the freedom of options available to a system as it evolves, just as friction or an inclined plane limit or constrain the speed of a moving object (Hooker, 2013). I argue that emotional episodes can be differentiated from ongoing psychophysiological homeostasis during periods of time in which emotion parts appear to constrain each other.
I then suggest that if emotions operate via mechanisms of constraint, we need to consider new approaches to measuring emotional synchronisation (Section 5), ones that align with a construal of emotions as emergent properties. It is not my purpose to outline exactly what these methods ought to be. Instead, I offer a few tentative suggestions and explain why they make sense in context of the explication of emotions as emergent properties provided here. In particular, I explain that understanding emotions with reference to constraints demands seeing tokens of a given emotion type as sharing a family resemblance,5 rather than being identical across contexts (e.g., not all instances of anger will have an identical ‘signature’ across persons and situations). This is because the causal factors (discussed in Section 5) that will continuously influence such a system will prevent any emotional patterns produced from conforming strictly to an idealised type. It is hoped that this approach to construing emotions as emergent properties may inspire new approaches to measuring the presence of emotional ‘signatures’, while also functioning as a new case study supporting the value of ‘pattern emergence’ as a powerful vehicle for characterising the objects of investigation — compound and context-sensitive — ubiquitous in the biological sciences.
2 Dynamical patterns
The proposal that emotions are emergent phenomena is by no means common or well-accepted in emotion theory. Rather, it features in the work of a small body of theorists with an overarching interest in the temporal dynamics of emotional episodes as they unfold over time. Those who adopt a dynamical approach to characterising emotion sit broadly within what have been called enactivist (Scarantino & Sousa, 2021) and ‘network’ (Moors, 2022) approaches to emotion. These approaches do not neatly oppose approaches that see emotions as feelings, as judgments, as perceptions, or as motivational states (see Scarantino & Sousa, 2021, for a typology and discussion of these positions). Rather, the difference lies in explanatory focus: Those adopting a dynamical approach are less interested in identifying the core feature or features of emotional states, but rather in using concepts and tools drawn from dynamical systems theory to help describe how the component parts that make up emotional episodes change across time, with the ultimate goal of improving our knowledge of the mechanisms that produce, sustain, and dampen emotional episodes.
A much-discussed feature of dynamical systems is that they can give rise to emergent properties. In the context of emotion, the dynamical system is hypothesised to be identical with most subsystems of the human organism, or the human organism itself (see Meuleman, 2015, for discussion), and emergence is defined specifically in relation to the formation of emotional patterns (that is, patterns that form over time). Colombetti (2014, p. 77), for instance, describes emotional episodes as ‘dynamical’ or ‘coherent’ patterns that form against a background of what she calls ‘primordial affectivity’ or ‘sense-making activity’ (Colombetti, 2014, pp. 1–24). The notion of primordial affectivity has some overlap with the idea of ‘core affect’ described by psychological constructionists (e.g., Russell, 2003) as the ‘bare’ or ‘raw’ feeling of bodily arousal, prior to conceptualising, verbalising or labeling. However, Colombetti’s (2014, p. 19) notion of primordial affectivity, drawn from the enactivist and phenomenological traditions, is broader, denoting a very primitive sort of teleological directedness, i.e., the ability that even very simple living systems have to ‘be sensitive to what matters to them’. An example of this in a simple system is the movement of a motile bacteria toward a sugar gradient.
A crucial feature of this approach, then, is that emotional episodes do not form against an otherwise non-affective background. Instead, emotional episodes are ‘coherent patterns of an organism that is always already affective in virtue of its being a sense-making system’ (Colombetti, 2014, p. 77). For Colombetti, emotional episodes have distinctive configurations not because of the preformationist assumptions espoused by basic emotions theorists (i.e., Ekman et al., 1972; Ekman, 1973, 1980) but rather in virtue of a history of mutual influences between evolutionary and developmental factors that shape the phenotypic trajectory of the organism. On this ‘softly assembled’ approach (see especially Colombetti, 2014, pp. 69–74) all emotional episodes, including those associated with basic emotions, are open and flexible structures sensitive to context.
The identification of emotion with dynamical patterns also appears in component process models of emotion. For instance, in the component process model (CPM) of Scherer (1984, 2009b, 2009a), emotion is a process of coordination or synchronisation of emotion components, driven by appraisal from a relatively uncoordinated or loosely coupled state. Here, the concept of synchronisation is used to separate business-as-usual system operations from distinctively emotional patterns. It is only when a minimum threshold of synchronisation is achieved amongst components that an emotional episode begins. The emotional episode is identical with the ongoing interactions of the emotion components over time until synchronisation falls below the minimum required threshold. Emotion is described as emergent here (Scherer, 2009b, 2009a) in the sense that the emotional episode forms a pattern that emerges over time. That this pattern appears is clear if we imagine an emotion appearing and disappearing, in a multitude of infinitely varied formations, on a state space that tracks how the interaction of components forms attractor basins. Each attractor is a visual representation of the emergent pattern formed by each emotional episode. The emergent phenomenon here simply is the pattern, identical with the emotion itself. Though the accounts of Colombetti and Scherer are distinctive,6 both share the broad idea that emotion component interactivity produces patterns of ‘synchronisation’ or ‘soft assembly’, and that these patterns differentiate emotional from non-emotional episodes.
3 Emergent dynamical patterns
Though Colombetti and Scherer both identify emotional patterns with ‘emergent’ emotional episodes, neither attempts to show whether and how these patterns meet the two-fold criteria for emergence introduced above:
- Determination:
- E is determined by B.
- Novelty:
- E is novel with respect to B.
I now turn to this task. These criteria can be cashed out in various ways, corresponding to different types of emergence, and it is not my intention to provide a comprehensive survey here (existing taxonomical approaches are discussed in Guay & Sartenaer, 2016; Humphreys, 2016; Sartenaer, 2016). Instead, I focus on what has been called ‘pattern emergence’ (Humphreys, 2016; Winning & Bechtel, 2019), an approach that aligns emergent phenomena with the formation of patterns produced by the dynamical activity of complex systems, and is thus suitably paired with the case of emotional patterns understood as putatively emergent phenomena.
Note firstly that, on a pattern emergence approach, the determination condition is met because the emergent whole E and its base B are in a constitutional part-whole relationship. Across the period of time an emotional episode is present, the parts change along with the whole (for discussion of this conception of diachronic constitution, see Craver & Bechtel, 2007). For example, during an episode of anger, there is typically a sharp increase, followed by a gradual decrease, in heart rate and nervous system arousal, concomitant with rumination on the theme of blameworthiness. This is the sense in which the emotion as a whole is constituted by its parts. Now, it is true that these components are present when an emotional episode is not (heart rate can increase even if we are not feeling emotion, for instance, and rumination can occur without anger). The difference for our purposes is that identifying the emotion requires recourse to a different form of description: We cannot identify the presence of an emotional episode simply by describing the current state of the various parts making up the emotion, or even by providing values for variables associated with each of these parts. This is because, on a dynamical approach, we are looking for a relationship of temporary synchronisation that holds among the parts when represented as variables in a time-dependent statistical model. As such, the pattern that constitutes the emotion can only be described using an alternative level of description.7 In practice, this alternative level of description will include terms referring to statistical measures of synchronisation (such as a moving principal component analysis or PCA, see Hollenstein & Lanteigne, 2014), as well as terms that describe the strength of interactivity between the component parts (feedback loops and constraints are examples of such terms, and are discussed in detail below).
We can now turn to the novelty condition. Some varieties of emergence focus on the generation of ontological novelty, such as new properties, laws, or causal powers that arise with E (these can arise either synchronically, diachronically, or both, see Guay & Sartenaer, 2016). Other approaches focus on epistemological novelty, where E is a novel representation of the relevant system that is unpredictable, unexplainable, or impossible to describe when armed only with B-level information (this sort of novelty can also occur either synchronically or diachronically).8 Novelty on a pattern emergence approach is sometimes cast as epistemological and diachronic (Humphreys, 2008, 2016). Here, the focus is on an emergent pattern E whose formation is impossible to predict at time t2 when armed only with information about B at time t1. However, it has also been argued that the unpredictable, emergent pattern also produces a form of ontological novelty. A proposed example of the latter is the emergence of ‘goal-directed behaviour’ in complex and adaptive biological systems (Winning & Bechtel, 2019). The possibility that emotion may be ontologically emergent in this sense is an interesting one, but I focus below on epistemological novelty cast as unpredictability, since this will, as we shall see in Section 6, prove useful for thinking about the boundary problem (introduced in Section 4).
We will be in a better position to characterise the sort of unpredictability we are faced with in the case of emotion by considering a simple example. Imagine a student who feels fear at t2 when called on to speak in class. Component changes associated with fear occur: The student adopts the facial expression associated with fear (wide eyes, raised eyebrows) and concomitant physiological changes occur (e.g., raised heart rate, respiration, and blood flow, see Hyde et al., 2019). The prediction of what emotion the student will feel at t2 cannot be made going merely on measures of basal-level components (the student’s facial expression at time t1, heart rate at time t1, and so on). Since knowledge of B-level markers at t1 do not enable knowledge of E-level markers at t2, the criterion for unpredictability is met in a rather minimal sense.
However, this notion of unpredictability might be thought too weak to classify emergent phenomena (for discussion see Sartenaer, 2016). How does it differ from, say, the difficulty of predicting if a car will speed up at t2 when armed only with knowledge of the state of the engine at t1? Without knowing that the driver has pressed on the accelerator pedal at t1, we cannot predict E (acceleration) at t2. And we presumably would not want to say that acceleration is an emergent property of an engine, lest we risk trivialising the notion of emergence.9
There is, nonetheless, an important point of contrast between the case of acceleration as a putative emergent property, and the case of emotion. The function of a car engine (e.g., what elicits acceleration, how much acceleration is produced when pressing on the pedal with a certain degree of force) is determined when the engine is designed. Its mature function is not a product of an adaptive developmental history unique to each car engine. But the case of emotion most likely is. Consider firstly the fact that human emoters respond very differently to identical environmental input. This is so both with regard to the initiation of an emotional episode, and its regulation (how it develops, and what leads it to either continue or terminate). Consider initiation first: Being cut off in traffic on the freeway may prompt mild annoyance in one person, rage in a second person, and may inspire no emotional change in a third (imagine a third person who notices being cut off but continues enjoying the view of the water over the bridge while listening to the radio). A gentle dig might amuse one person, make a second person feel angry, and make a third person feel sad. Now consider regulation: Out of two people who respond with anger to being cut off in traffic, or the mildly insensitive dig, one might quickly return to a state of relative emotional neutrality, while another might remain angry for minutes (or hours, or perhaps even days). This variation in emotional elicitation and expression has been dubbed the ‘singularity problem’ (Morag, 2016).
It is likely that this variation is due to developmental learning. Consider, for instance, the tendency to respond to environmental input with anger. Influential work by Dodge & Somberg (1987) suggested that repeated experiences of abuse, such as aggression and/or violence lead children to develop a ‘hostile attributional bias’ (HAB) that filters subsequent experience. Dodge claimed that those who make hostile attributions will, when exposed to a frustrating social stimulus, such as being hit in the back with a ball, tend to interpret the stimulus as an aggressive cue and thus respond aggressively. Dodge & Somberg (1987) called this a sort of habitual ‘cue distortion’ (also see Anderson & Graham, 2007), one that manifests as dispositional anger that ignites or persists even when outer circumstances do not seem to warrant it. Development of HAB might explain cases of variation in emoting in which individuals respond with anger to a neutral stimulus.
One benefit of a dynamical approach to emotion is that it offers concepts and modelling techniques that can be used to represent how emotional dispositions are formed over developmental time (especially in childhood and adolescence) and come to influence how occurrent emotional episodes are triggered and expressed in a given individual (for discussion, see Lewis, 2005; Lewis & Liu, 2011; Walsh, 2021). And if it is indeed the case that the triggers and dynamical trajectories associated with emotional episodes are influenced by an individual’s life history,10 E at t2 will prove unpredictable even when we are armed with knowledge of B at t1 and knowledge of an observable trigger (such as a teacher calling on a student to speak, or a person being unexpectedly hit on the back with a ball). We could certainly make educated guesses in these cases, but we will be much less certain of our predictions than in the case where we try to predict that a car will accelerate with knowledge of an observable trigger (pressing on the accelerator pedal). The difference is that emotions are flexible by design: unlike blinking when an object is suddenly moved close to the eye, emotions do not unfold in the same way for all people when faced with similar triggers. This is a well-documented feature of emergence in complex adaptive systems (Humphreys, 2016; Winning & Bechtel, 2019), and it is in this stronger sense that E is unpredictable in the emotion case.
4 The boundary problem
So far, I have described emergent emotions as patterns produced by a system at t2 that are unpredictable when armed only with knowledge of the system set-up and any relevant environmental triggers at t1. This definition presupposes that we can clearly distinguish a pattern from a non-pattern. But how do we tell the difference between ‘synchronised’ emotion components and ongoing homeostatic processes? This is, as was explained in Section 1, the boundary problem. Emotion theorists who have tried to measure synchronisation have sought emotional ‘signatures’ for given emotion categories (anger, fear, disgust, etc.). These ‘signatures’ refer to covariational patterns among one or more emotion components that identify tokens of a particular emotion type.
Whilst there have been efforts to detect emotional signatures, the consensus view is that the empirical evidence provides either weak or no support Lench et al. (2011). However, these studies are limited in at least two significant ways. First, many only measure covariance between two components, with few measuring covariances among three or more components of emotion (Barrett, 2006a; Meuleman, 2015). Second, the measured covariances are assumed to be static (meaning covariation is not indexed to time, but is instead presented as a summary state at a single moment in time, see Meuleman, 2015).
Having said this, recent work on emotional synchronisation has begun to identify time-indexed covariational patterns among emotion components (Hollenstein & Lanteigne, 2014). Some of this work uses multivariate analysis of variance (MANOVA) across designated time slices and uses various psychological and physiological measures as proxies for changes in emotion components. Such work is in its infancy but has found significant evidence showing feedback between multiple emotion components (for discussion and examples of recent empirical work, see Bulteel et al., 2014; Hollenstein & Lanteigne, 2014). In addition, methods for collecting and dynamically analysing multi-componential, time-indexed data are developing in sophistication, and dynamical analyses of various emotional phenomena are being used with increasing frequency (see Kuppens & Verduyn, 2017). Unfortunately, however, there are not yet studies using specifically nonlinear, time-indexed methods to specifically assess multi-component emotional synchronisation (see Meuleman, 2015).
In Section 4, I explore the idea that constraints (feedback loops between emotion components) constrain or reduce the output options available to the system as a whole during the period of time for which an emotional episode is present. Feedback loops are, as we shall see, temporal phenomena. It is also well-recognised that capturing their dynamics requires the use of nonlinear methods (I return to this point below, see also Lucky et al., 2020; Scherer, 2009a). If emotions are inherently temporal phenomena that operate via feedback loops, we should expect that static and linear statistical methods will be unable to reveal their presence. I now discuss how feedback loops are involved in emotion production, and then show how the notion of emotions as emergent patterns provides the conceptual backdrop that motivates and justifies the application of nonlinear dynamical analysis to the search for emotional signatures.
5 Constraints
In classical physics, laws governing motion characterise the way a system might evolve, and constraints limit the degrees of freedom available to the system as it evolves, just as friction or an inclined plane limit or constrain the speed of a moving object (Hooker, 2013). A simple example of a physical constraint is a bowl that constrains the movement of a marble. The marble can move in any direction, but only as far as the edges of the bowl. Another example is a skeleton, described by Hooker (2013, p. 761) as a ‘disabling constraint, for example limiting the movements of limbs (cf. an octopus)’. An octopus limb has more ‘degrees of freedom’ with respect to forming curves than a human arm, for instance, since the latter can only bend rigidly at the elbow.
A number of authors have applied the notion of constraint to feedback loops in biological systems (Bechtel, 2017; Hooker, 2013; Pattee, 1971; Winning & Bechtel, 2018). Here, feedback loops produce a constraining effect by amplifying or dampening the output of a mechanism over time. A feedback loop is a system where outputs at time t-1 are routed back as system inputs at t. An ‘output’ refers to any variable that can be measured, and that is part of the phenomenon being investigated. When a variable is described as an ‘output’ rather than a variable, this denotes the value that a variable (such as heart rate) is taking at a particular moment in time t of interest to the observer of the system (rather than indicating an output to system activity considered as separate from the phenomenon itself). A positive feedback loop features outputs routed back as inputs that tend to amplify the strength of the incoming signal, or the level of perturbation of the system. (A simple example is a bank account with compound interest. Deposits into the account increase the total balance, and compound interest amplifies this effect). A negative feedback loop produces the opposite effect, dampening or weakening system perturbation. Below, I rehearse Bechtel’s (2017) example of a feedback loop operative in the context of circadian biology, to illustrate the relationship between feedback loops and output options available to a system. This is followed by an example of just one feedback loop (between arousal and appraisal) that is likely involved in the case of emotion, and that also affects system outputs.
Consider how the notion of constraint can be used to characterise feedback control in circadian biology (Bechtel, 2017; Hardin et al., 1990). In particular, consider the relationship between oscillatory phase and the lower-level parts of the mechanism (the genes, proteins, etc.). It is now known that entrainment to light comes when a light signal from the retina to the suprachiasmatic nucleus functions to increase transcription of the Per gene (Bechtel, 2017). The function of light on transcription varies depending on the time of day. During the day, light has no effect on transcription because Per transcription is already at its maximum. During early evening, light exposure delays the phase of the oscillation. This is because, early in the evening, Per concentration diminishes due to an internal feedback mechanism. Late at night however (e.g., approaching dawn) light exposure advances the phase. This is because during this time Per concentration is beginning to increase due to endogenous oscillation. As such, light received during this phase will speed up transcription, resulting in daytime levels of the PER protein being reached earlier, and an advance in the phase of the oscillator.
The important point here is that circadian rhythm can be construed as a mechanism whose output varies even when the type of input (i.e., light) remains constant. Sometimes the one input type will increase Per transcription, and other times it won’t. What this variation in output depends on are feedback mechanisms internal to the mechanism itself, such as feedback about the concentrations of the PER protein in the nucleus: When PER levels are low, synthesis of new PER proceeds, but as PER accumulates, it inhibits further synthesis. The product is a system that responds differentially to the same input. The effect on Per transcription during, for example, the state in which PER concentrations in the nucleus are high is determined by conditions generated within the mechanism, not from outside it.
This system-dependent differential output is produced by constraint. This is so in the sense that the mechanism operates with reduced degrees of freedom, meaning that the total possible output options of the mechanism is reduced. For example, feedback about the concentrations of the PER protein in the nucleus can reduce output options such that, if Per transcription is increasing, the system will tend toward more quickly reaching daytime-typical concentrations of PER. No longer is there the option for concentrations of PER to increase or decrease. In this case, output options are reduced to those consistent with an increase in PER concentration.
Below, I suggest that we can apply the notion of constraints to emotion. In particular, feedback loops are thought to be involved in emotion (see Hollenstein, 2015), and I suggest that we see them functioning to reduce the output options available to a system, where here the ‘system’ is an individual reacting to their environment. To make the point, I consider a very simple case of one feedback loop – between arousal and appraisal – that is likely involved in the emotion of anger. I first briefly summarise the evidence base relating these components in the case of anger, and then provide an example to illustrate why the notion of constraint is useful.
To start with, there is evidence that heightened positive functional connectivity with the thalamus during angry rumination reflects a reciprocal relationship between rumination and arousal (Denson, 2013). The thalamus is a key structure in the brain that acts as a relay station, receiving sensory information from various parts of the body and relaying it to the relevant brain regions for further processing. In the context of angry rumination, the heightened positive functional connectivity with the thalamus indicates an increased level of communication between the thalamus and other brain regions involved in executive functions, such as the prefrontal cortex. This suggests that the executive functions associated with the prefrontal cortex (e.g., planning revenge), co-occur with an increased sense of arousal.
There is further support for the idea that there is reciprocal influence between arousal and appraisal. Consider firstly how arousal influences appraisal. There is growing support for the view that arousal motivates and influences reasoning processes. For instance, Haidt (2001, 2013) showed that a person’s ‘gut reaction’ to a vignette appeared to drive reasoning processes in a biased way: toward a reasoned assessment of the situation described in the vignette as conforming to the initial feeling the vignette provoked (e.g., get angry first, justify your anger later). More recently, it has been suggested that the appraisals we develop in response to environmental input (and, consequently, the way in which we ruminate about such appraisals) may be influenced by our interoceptive awareness of physiological changes in the body (Miller & Clark, 2018; Wilkinson et al., 2019).
Now let’s consider how appraisal influences arousal. It is known that the perception of physiological activity, otherwise known as interoceptive awareness, can heighten the intensity of emotional arousal (Dunn et al., 2010). Consistent with the role of the insula in interoceptive awareness, Denson et al. (2009) found, for instance, that right anterior insula activation is positively correlated with self-reported angry state rumination. Thalamus activation is also important due to its role in emotion processing, emotion experience, and emotional control (Marchand, 2010). Together, increased activation in the right anterior insula and thalamus during rumination may be associated with a heightened experience of emotional arousal (see also Denson, 2013).
We can now consider an example (represented in figure 1 below). Imagine a high-school art student working on a painting in class. At time t1, she is in a relatively unemotional state. Her nervous system is unaroused, and she is daydreaming vaguely about various topics (what she will buy for lunch from the canteen, whether she will get an A in this class, etc.). At t2, her desk partner accidentally elbows her as he switches paintbrushes. The first student immediately experiences physiological arousal prompted by the unexpected pain in her arm (e.g., increased cardiac output and sympathetic nervous system arousal, see Sinha et al., 1992). She then looks at her classmate’s facial expression, which is ambiguous, somewhere between shock and surprise. Due to her aroused state, however, she interprets this expression as hostile, developing an appraisal consistent with the thematic content of anger, namely that a ‘demeaning offense against me or mine’ has occurred (Lazarus, 1968; Silvia & Warburton, 2006). At t3, the student’s appraisal that her desk partner intended to hurt her now feeds back to stimulate further physiological arousal (her heart rate increases further, and her cheeks begin to redden in the familiar flush of anger). At the same time, her increased arousal stimulates and sustains a developing appraisal of her classmate as a demeaning bully deserving of blame or retaliation (at this point, perhaps she scowls at him or demands an apology). At t4, interoceptive awareness of her state of sympathetic arousal further constrains the student’s evaluation of the event (her classmate’s putatively genuine apology is met with resistance, and she gets up and abruptly leaves the classroom, ruminating on her classmate’s vicious attempt to ruin her art work).11
In this example, a positive feedback loop between arousal and appraisal operates to generate and sustain an instance of anger. The feedback loop acts as a system constraint in the following sense: At t2, physiological arousal results in the student developing an initial appraisal consistent with anger (e.g., that of a demeaning offence). Whereas at t1, the student was thinking idly about various topics, at t2 she only has a single point of focus, namely the idea that her classmate intended to hurt her. This appraisal then constrains her state of arousal (her nervous system continues to move toward heightened sympathetic arousal, consistent with the development of anger). Finally, at t4, heightened arousal and an angry appraisal remain tightly coupled, as the student abruptly leaves her desk so she can ruminate on her classmate’s apparent wrongdoing.

Figure 1 above provides an illustration. Circles represent appraisal (grey) and physiological arousal (white). The circle size represents the flexibility or degrees of freedom available for each variable during successive moments in time (with a smaller size indicating a reduction in degrees of freedom). Arrows represent positive feedback between arousal and appraisal. Decreasing circle size shows a decrease in flexibility as a result of reciprocal feedback between arousal and appraisal. Though the episode of anger is initiated by an environmental stimulus, it is developed and sustained by the student’s continuing rumination and concomitant arousal. This process results in a reduction in the range of values that may be taken by measures of arousal and appraisal during the episode of anger. This result manifests in the student’s sharp focus on the blameworthiness of their classmate (instead of her thinking about the situation in a more flexible and open-ended way), and in her heightened sympathetic nervous system arousal (instead of experiencing a variable heart rate, for instance, the student’s heart rate instead increases steadily as she continues to ruminate on the blameworthiness of her classmate).
6 Flexible boundaries
I now want to suggest that if emotions operate via mechanisms of constraint, we need to consider new statistical approaches to measuring emotional synchronisation. It is not my purpose here to outline exactly what these methods ought to be. Instead, I offer a few tentative suggestions and explain why they make sense in context of a pattern emergence approach to emotion. Finally, I explain how the novelty condition is met on this new approach.
First of all, note that in our simplified case involving anger, we saw that the output options available with respect to arousal and appraisal are reduced to those consistent with anger (e.g., increased cardiac output, rumination consistent with the theme of other-blame) and that it is the presence of feedback between arousal and appraisal that helps to develop and sustain the emotional episode. I characterised this feedback as a constraint on the system that left it operating with reduced degrees of freedom. Despite this, constraints do not completely fix or determine how a dynamical episode of anger will develop and sustain (or resolve) itself across time: Constraints reduce the degrees of freedom available to the system when an emotional episode is occurring, rather than fixing them completely. Let me describe two other influences (separate to the constraints) that influence the dynamical pattern constituting the emotion.
Firstly, ongoing changes in the material and social environment will modulate the development of the emotional episode, as will internal homeostatic processes that do not feature as emotion components in a given statistical analysis. Both processes can be seen as ‘control parameters’ – factors that necessarily affect the state of the system, but are not necessarily in turn affected by the system. An example of an environmental change is a social interaction providing negative feedback that dampens the intensity of the emotional state. For instance, perhaps at t5, a reassuring hug and a soothing word from a friend in the hallway interrupts the student’s narrow focus on blaming her classmate, and she begins thinking of alternative interpretations of her classmate’s behaviour. At the same time, her heart rate begins to decrease (see figure 2). Here, external input into the system acts as negative feedback, dampening the student’s emotional response. (Without positive feedback, emotional episodes could not reach a finite zenith, and without negative feedback, they would never resolve into neutral or less intense states.) An example of an internal homeostatic process influencing the system is an existing case of hypertension that exacerbates the student’s interoceptive awareness of her increased cardiac output (see Yigla et al., 2006) associated with a surge of anger.

Importantly, these ongoing changes, represented as control parameters, may only be visible when emotions are modelled as nonlinear, time-dependent functions. A linear function is such that any change in an independent variable x (e.g., x could be an emotional stimulus, such as a scene from a scary movie) will always produce a corresponding change in a dependent variable y (e.g., y could be a physiological or expressive change indicative of fear). In this way, a linear function is predictable. But the control parameters just described are, in their essence, unpredictable. Therefore, we should not expect that traditional linear statistical analysis (of the sort typically used to measure emotional synchronisation) will reveal the presence of emergent emotional patterns.
The second issue is that these ongoing environmental changes may not only influence how an emotional episode develops and sustains itself across time, but whether or not a given covariational pattern ‘counts’ as a token of a given emotion type. Consider again the case of the hypertensive individual. The threshold for physiological markers of the presence of anger could be expected to differ in experimental settings featuring hypertensive patients versus those featuring non-hypertensive patients (since hypertension increases cardiac output, which is frequently used as a proxy indicator for anger-related arousal). Furthermore, it may be that measures of covariance that are statistically significant may be an intra-individual phenomenon, such that the more we try to generalise across persons, the more fragile the generalisations drawn become. Therefore, we might be asking for too much if we seek a single, context-independent measure. Instead, we might need to seek measures that fall within a range of indicators for statistical significance, with each range relativised to a specific context of inquiry.
I close with a few words about how the notion of pattern emergence may encourage us to be more flexible with respect to the search for emotional signatures. As was noted earlier, the distinctive feature of novelty on a pattern emergence approach is that the emergent pattern E produced at t2 is unpredictable when armed only with knowledge about the basal-state B at t1. We are now in a position to further clarify the notion of unpredictability at play here.
Firstly note that there is no need to assume that the emerging pattern at t2 has some sort of essential property, or even that it conforms precisely to some or other category type or definition (see Humphreys, 2016 for discussion). It is sufficient that the pattern be unpredictable and historically related to the basal-level configuration at t1. To make the point clearer, consider the ‘bow-tie’ shape produced by a cellular automaton following a set of rules that determine how its states evolve over time (figure 3 below). The condition for the ‘bow-tie’ being an emergent pattern is that it is produced by the evolution of the cellular automaton over time. If the pattern is produced by, say, creating a matching stamp and stamping it on to a piece of paper, it does not count as an emergent property (for discussion see Humphreys, 2008).
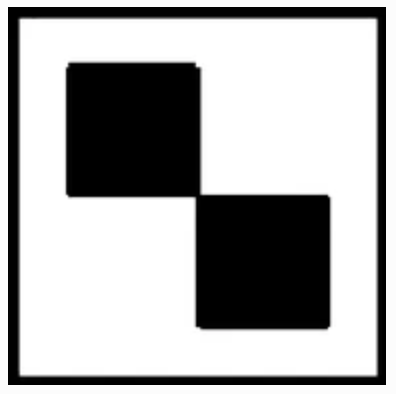
If emotions are novel in this historical, system-dependent sense, we have an additional reason not to demand that tokens of emotion conform to an ideal or essentialised type. This is that emergent patterns are principally defined by their unpredictable emergence in the life of a given system. There need not be an additional requirement that the pattern produced conform to a strict type identity.
Nonetheless, this does not mean that it is impossible to identify broad commonalities among all emotional episodes of, say, anger, or fear, or disgust as they begin to emerge. I have argued that the notion of constraint can explain why emotions appear to occur over discrete periods of time, in opposition to, say, moods, or periods of relative non-emotionality (e.g., that a sudden flash of anger, say, seems to have a discernable time course, concomitant with flushed cheeks, a raised voice, and an increased heart rate). The explanation is that the dynamical system (identifiable with the human organism itself) is constrained when an emotional episode is present such that it operates with reduced degrees of freedom. An emotional episode will produce a pattern whose exact configuration is unpredictable in any specific case, but which, when compared across persons and situations, will exhibit broad configurative similarities that can be described with approximate covariance measures. The degree to which such a patterned identity emerges will reflect the degree to which the system is constrained.
7 Conclusion
This article explored what it might mean to characterise emotions as emergent properties, building on existing proposals to construe emotions as dynamical patterns whose emergence is unpredictable in the life of a given system (e.g., a given emoter). It proposed aligning the view that emotions are emergent properties with a ‘pattern’ emergence approach in which novelty is cast as epistemic unpredictability. It also argued that the emotion mechanism as a whole functions to reduce the degrees of freedom of its various components, via the operation of constraints (realised via the feedback loops characteristic of nonlinear dynamical systems). It might be hypothesised that the operation of these constraints, and the patterns to which they correspond, themselves appear at the ‘edge of chaos’12 — at the boundary between the random behaviour of a complex system and a self-organised state (Humphreys, 2016; Packard, 1988).
It has been recognised that one of the distinctive features of the nature of explanation in the special sciences is seeking generalisations that hold under a relevant class of interventions, rather than exceptionless laws (for discussion see Woodward, 2000, 2007). A characterisation of emergence as constraint suggests that previous attempts to identify emotional signatures may have been in search of a chimera — a precise, lawlike and contextually-invariant signature for any given emotion type. Construing emotions as emergent properties in the manner proposed here offers an alternative to pre-emptively abandoning the task of seeking emotional signatures for at least some emotion categories. Instead, emotional signatures might be hypothesised to have measures that fall within specified ranges, that feature inter-individual variation, that are context-sensitive, and that are identifiable with patterns only visible under the lens of dynamical systems analyses. Such measures would be ‘invariant’ in the sense described by Woodward (2000): they would hold under a relevant class of interventions, even if not exceptionlessly beyond that domain. Seeking such measures yields an approach to empirical investigation consistent with the nature of generalisations distinctive of the special sciences — limited and more fragile, but not non-existent.
Acknowledgments
Some of the ideas in this paper were presented to members of the Centre for Technomoral Futures at the Edinburgh Futures Institute, University of Edinburgh. These ideas have evolved from research that began whilst working within the Theory and Method in Biosciences Lab at the Charles Perkins Centre, University of Sydney. I received valuable feedback from both groups. I also received instrumental feedback from Laura Kotevska at the University of Sydney, and from two anonymous reviewers who provided assistance in refining the central argument of the paper. I am thankful for the generous support for this research provided by the University of Wollongong’s AEGiS Renew Grant Scheme (2022-2023), and for the support of my colleagues at the School of Liberal Arts, University of Wollongong.
References