1 Introduction
There are clear limitations on our ability to control our own thoughts. It is very challenging to focus perpetually on a particular thought (Pashler et al., 2001), to avoid a particular thought (Abramowitz et al., 2001), or to stop thought altogether (Woods et al., 2024). Control over moment-to-moment mental content is highly variable within and across individuals (Feliu-Soler et al., 2019). People might spend up to a third of their day off-task, thinking of topics they had no intention of thinking about (Kane et al., 2007), and even more under times of stress (Smallwood et al., 2009). Furthermore, the structure of thought streams appear altered in many clinical populations, including ADHD (Alperin et al., 2021; Van Den Driessche et al., 2017) and frequent nightmare sufferers (Carr et al., 2016). Despite large fluctuations in thought trajectory and the clinical relevance of such fluctuations, neurocognitive features that influence thought trajectory are still largely unknown (Mildner & Tamir, 2019). Understanding such features is expected to lead to refined models of cognition and subsequent treatment options.
The Dynamic Framework of Thought is one theoretical framework that has attempted to describe the flow of thought across the sleep-wake cycle (Christoff et al., 2016). In this framework, various mental states are arranged in a two-dimensional space where constraints from various sources play a large role in their level of spontaneity. Cognitive control is one of the two main sources of such constraints according to the Dynamic Framework (the second source is automatic constraint processes). Lower cognitive control leads to more spontaneous thoughts in waking (i.e., mind-wandering) and sleep (i.e., dreaming). Within this framework, thoughts that are more “goal-directed” (and therefore associated with greater cognitive control) are predicted to have a narrower focus (and thus less semantic variability) compared to thoughts that are less deliberately constrained, which may represent times when the mind is wandering from one thing to the next.
Despite this clear theoretical prediction, the Dynamic Framework of Thought has not been widely empirically tested, particularly within the context of two mental states that purportedly have higher variability in thought: mind-wandering and dreaming (Christoff et al., 2016). Furthermore, most prior work investigating cognitive control’s relationship with thought treated waking and dreaming as uniform or categorical states. Thus, whether cognitive control has a similar influence on thought variability in both dreams and wake of a healthy population is still unknown. Studies are needed to address this question in order to test the Dynamic Framework and to situate spontaneous thoughts across the sleep-wake cycle within a clearer and more continuous dimensional space. A more explicit understanding of how spontaneous thoughts arise, unfold, and move throughout the circadian cycle is critical for the future development of cognitive models.
Moreover, the difficulty of quantifying thought trajectory raises challenges for making progress in this research area. Self-report approaches include asking participants to push a button during thought transitions (Li et al., 2021) or to respond to a simple query about whether the mind was moving (Alperin et al., 2021; Brosowsky et al., 2021; Gross et al., 2021; Kam et al., 2021; Mills, Raffaelli, et al., 2018; Mills et al., 2021). The latter method has been used to dissociate thought trajectory from the broader concept of task-unrelated thought (Brosowsky et al., 2021; Mills, Raffaelli, et al., 2018). Another fine-grained approach is to have external raters evaluate a thought report for the number of hard transitions or jumps between thoughts (Li et al., 2021; Raffaelli et al., 2021; Sripada & Taxali, 2020). This process, though cumbersome, has led to the validation of powerful automated tools that quantify thought variability at larger scales and with more objectivity (Li et al., 2021; Sripada & Taxali, 2020). Such automated approaches leverage latent semantic analysis to extract the semantic embedding of individual thought segments and quantify the mathematical distance between consecutive thoughts in a high-dimensional semantic space (Li et al., 2021, 2023). Another approach is to identify the amount of episodic detail within each thought (Genugten & Schacter, 2024) and quantify these shifts (Mildner & Tamir, 2024).
One route to studying cognitive control is through lucid dreaming. Most dreams occur with reduced levels of executive function or cognitive control, indicated most succinctly by the ongoing misperception of being awake. More specifically, this inability to recognize the dream state is theorized to result from hindered perceptual reality monitoring (Dijkstra et al., 2022). In contrast, during lucid dreams the dreamer accurately perceives the dream state as a dream while it is still ongoing (Baird et al., 2019; Tononi et al., 2024). Roughly half of the population reports having had at least one lucid dream in their lifetime, and about one quarter report experiencing lucid dreams once or more per month (Saunders et al., 2016). In addition to increased metacognition and self-reflection (LaBerge et al., 2018; Voss et al., 2013), dream lucidity is positively associated with a host of other executive functions, such as autobiographical memory recall (LaBerge et al., 2018; Voss et al., 2013). Dreamers often perform deliberate actions to influence the dream while lucid (Mota-Rolim et al., 2013; Stumbrys et al., 2014), including the performance of tasks that were planned prior to sleep (Dresler et al., 2011; Sandell et al., 2024) as well as in response to stimuli presented during sleep (Konkoly et al., 2021; Türker et al., 2023). Together, the broad increase in executive function and decision making that occurs during lucid dreams includes a general increase in cognitive control (Tononi et al., 2024).
In the current study, we hypothesized that conditions typically associated with relatively increased cognitive control in both sleep and waking would be associated with narrowed thought trajectories (i.e., reduced semantic thought variability), as predicted by the Dynamic Framework of Thought. We quantified thought variability in waking and dreaming thoughts that varied in their amount of self-reported cognitive control. Waking thoughts were reported throughout the day along with two distinct self-reported measures of mind-wandering: task-unrelatedness and the freedom of movement in thought (Mills, Raffaelli, et al., 2018; Mills et al., 2021). High and low cognitive control in waking were viewed as on- and off-task thought, respectively. Dreaming thoughts were reported in an online dream journal along with self-reported levels of lucidity (Schredl et al., 2022). High and low cognitive control in sleep were viewed as lucid and non-lucid dreams, respectively. In both these datasets, thought variability was operationalized as the semantic incoherence between moment-to-moment thoughts as they unfolded over time (Bedi et al., 2015; Corcoran et al., 2020; Elvevåg et al., 2007). Based on the Dynamic Framework of Thought (Christoff et al., 2016) and the Default Variability Hypothesis (Mills, Herrera-Bennett, et al., 2018), we expected that cognitive control would constrain thought variability, decreasing semantic incoherence (i.e., lowering thought variability) when cognitive control increased.
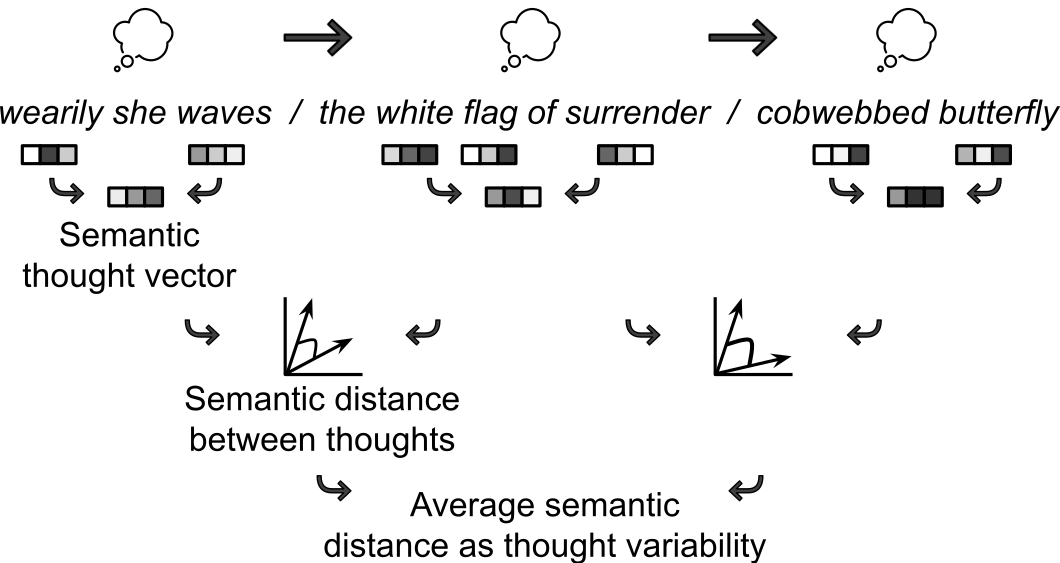
2 Methods
Our general approach was to aggregate subjective reports of waking thoughts and dreams under varying levels of cognitive control, to quantify the amount of thought variability within these reports, and to test the relationship between thought variability and cognitive control within wake and sleep. Datasets were selected from existing sources described below (Mills et al., 2021; Schredl et al., 2022), based on their wide coverage of different mental states.
2.1 Waking-thoughts dataset
To capture waking thoughts under variable levels of cognitive
control in daily life, we used experience samples from an ecological
momentary assessment study (Mills et al., 2021). Participants
reported their thoughts up to 6 times per day for one week in
response to text message prompts. Participants were told—upon
receiving a phone alert—to “take a mental snapshot of what they were
thinking about just before they saw the probe” and then answer some
questions regarding that mental snapshot. They were asked: (1) A
free-text description of their ongoing thoughts: “Please provide a
brief description of your most recent stream of thought based on the
mental snapshot.” (2) A report on task-unrelatedness, or whether
those thoughts were on- or off-task: “Are you thinking about
something other than what you are currently doing? Answer with
either a Y or an N.” (3) A report on a 6-point
Likert scale about freedom of movement in thought, or how much those
thoughts were freely moving: “Are your thoughts wandering around
freely? Answer on a scale from 1 (not at all) to 6
(very much so).” The second and third measures have been
used to assess mind wandering in previous studies and are tied to
constructs of constraints and executive control (Mills, Raffaelli, et al., 2018). See
Study 1 of Mills et
al. (2021)
for additional details regarding this dataset, including sample
information and additional measures that were collected. After text
preprocessing (see below), the final sample included
2.2 Dream dataset
To capture dreams (i.e., sleeping thoughts) under variable levels
of cognitive control, we used dream reports from a public online
dream journal (Dream Journals, n.d.; Schredl et al.,
2022). All dream-journal entries on this site include the
option to tag the dream as lucid and/or nonlucid. We started with
all dream reports where the author specified that the dream was
either only lucid or only nonlucid, and then reduced the dataset
further to only include authors who reported at least one or more of
both dream types. After subsequent text preprocessing (see below),
the final sample was reduced to authors who reported both
2.3 Semantic incoherence as a measure of thought variability
To quantify the amount of thought variability within a given text report, we calculated the amount of semantic incoherence of each text (Bedi et al., 2015; Elvevåg et al., 2007), as shown in Figure 1. This method relies on the extraction of semantic embeddings, which are numerical representations of words that capture their contextual meanings. These embeddings, or numerical vector representations, are derived from word co-occurrence patterns identified in large text corpora. In the current study, (1) each text report was segmented into individual phrases, (2) 300-dimensional semantic embeddings were extracted for each noun, verb, and adjective with four or more letters, (3) each phrase was summarized as the average semantic embedding of words within each phrase, (4) the cosine distance between each pair of consecutive phrases was calculated, and (5) the average of all cosine distances was the final measure of thought variability. Dream reports were segmented into sentence phrases, whereas mind-wandering reports were segmented into noun-chunk phrases (i.e., a noun and its surrounding context) due to their lack of clear punctuation or sentence structure. Any text without more than one phrase was removed. Text preprocessing, part-of-speech tagging, and semantic embedding extraction were performed using the spaCy Python package (Montani et al., 2023), where the language embedding space was trained on a large variety of English language sources including Wikipedia, news articles, and movie subtitles (spaCy en_core_web_lg model v3.4.1). Semantic incoherence thought scores were averaged within each participant and condition for all pairwise comparisons. Prior analyses have yielded a thorough validation of this method (Bedi et al., 2015; Elvevåg et al., 2007), justifying its extensive use in computational psychiatry (Boer et al., 2018; Corcoran & Cecchi, 2020).
2.4 Statistical analyses
All statistical analyses were performed using within-subject comparisons. A repeated-measures correlation was used to test the relationship between semantic incoherence and self-reported freedom of movement in thought during waking (Bakdash & Marusich, 2017). Semantic incoherence of on- and off-task thoughts were compared within subjects using a Wilcoxon signed-rank test. Semantic incoherence of lucid and nonlucid dreams were compared within subjects using a Wilcoxon signed-rank test. Effect sizes for pairwise tests were reported using the Common Language Effect Size (CLES) (Vargha & Delaney, 2000). All statistical analyses were conducted using the Pingouin Python package (Vallat, 2018).
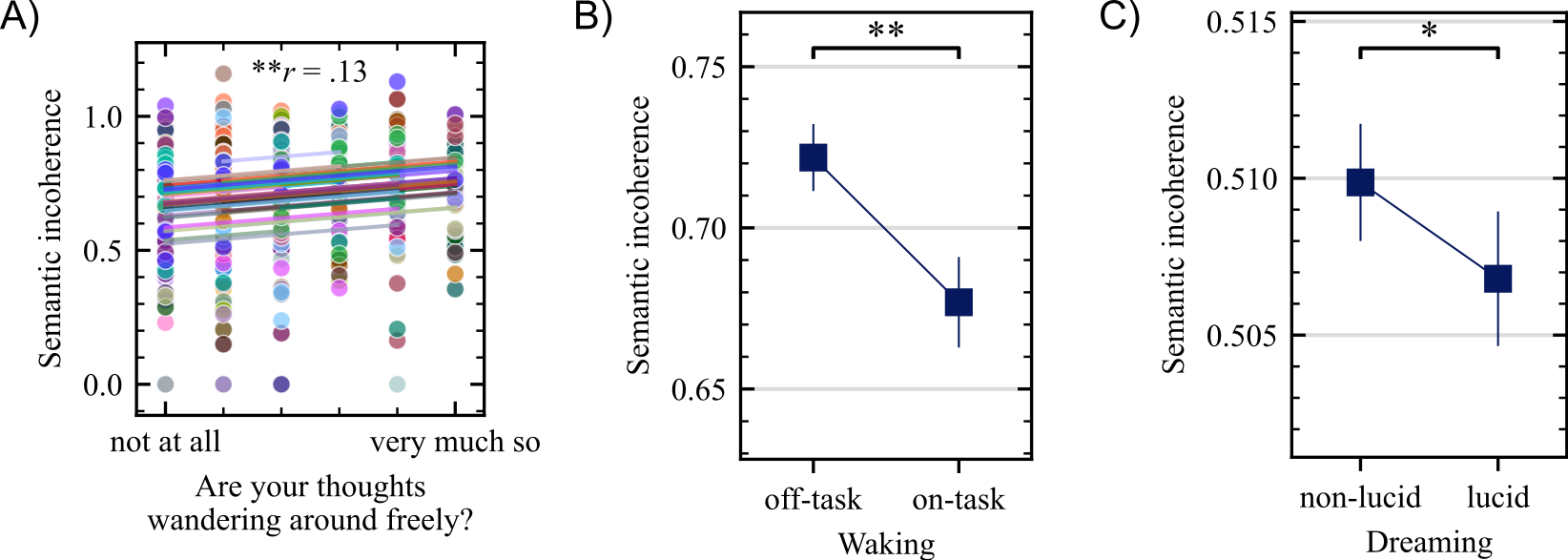
3 Results
3.1 Thought variability was reduced in wake when thoughts were on-task or wandered around less freely
We observed a positive correlation between self-reported freedom
of movement in thought and semantic incoherence (
3.2 Thought variability was reduced in sleep when dreams were lucid
We observed higher semantic incoherence scores (
4 Discussion
In the current investigation, we found that conditions typically associated with higher cognitive control were also associated with reduced semantic incoherence between moment-to-moment thoughts (i.e., thought variability). Reduced waking thought variability was associated with increased on-task thought and decreased levels of freely moving thought. Reduced sleep thought variability was associated with cognitive control while dreaming. By showing that conditions of increased cognitive control were associated with reduced thought variability in both sleep and wake, these results support the notion that cognitive control places a fundamental constraint on thought variability.
The continuous thought dynamics influenced by cognitive control within waking and dreaming are consistent with the Dynamic Framework of Thought (Christoff et al., 2016). This model places all thought on a continuum of deliberate cognitive constraints, which our data supports. Although prior work often references mind-wandering and dreaming as homogenous categories along the continuum of constraint, the current study provides support for sub-state degrees of deliberate constraints that operate within them (Mallett et al., 2021). A wide range of thought variability within and across both waking and dreaming might be driven by contextual demands and better serve a proposed function of spontaneous thought. For example, one potential function of spontaneous thought is to serve memory consolidation through episodic decoupling (Mildner & Tamir, 2019; Mills, Herrera-Bennett, et al., 2018). In this case, a wider range of thought variability within both waking and dreaming might allow for unique consolidation benefits that occur during periods of relatively high thought variability within each state.
Recent theoretical accounts place dreaming within a broad category of spontaneous thought (Christoff et al., 2016; Mildner & Tamir, 2019). However, our results suggest that lucid dreams, which are characterized by wake-like levels of cognitive control (LaBerge et al., 2018; Mallett et al., 2021; Voss et al., 2013; Windt & Voss, 2018), might be considered a unique case of relatively nonspontaneous thought during sleep. In the current study, lucid dreams showed reduced thought variability compared to nonlucid dreams, which resembled the reduction in thought variability from off-task to on-task thought while awake. Higher cognitive control during lucid dreams might allow the dreamer to engage in goal-directed and intentional thought. However, our analyses did not distinguish between lucid dreams with dream control and those without. Lucid dreams are often associated with having a degree of control over the dream narrative, but there are also instances of being aware of the dream with little or no dream control (Mallett et al., 2021, 2022), suggesting that dream awareness (i.e., lucidity) varies independently of dream control. Thus, it remains unclear whether awareness or control of the dream is more tightly bound to nonspontaneous thought.
Not only are dream control and lucidity separable, but each occur on a graded scale rather than categorically. In this study, we binned dreams into categorically lucid or non-lucid dreams based on the labels that authors provided in the dataset. However, prior work has spotlighted the continuous nature of lucidity in dreams, as well as how the extent to which dreamers can control their dream content or realize their intentions likewise varies along a continuum (Mallett et al., 2021; Solomonova & Carr, 2022; Voss et al., 2013; Windt & Voss, 2018). Previous studies have reported different estimates of the frequency and ease of dream control in lucid dreams, ranging from about 40% to 60% of lucid dreams (Mallett et al., 2021; Mota-Rolim et al., 2013; Schredl et al., 2018; Stumbrys et al., 2014; Stumbrys & Erlacher, 2017; Voss et al., 2012). Some of these studies also suggest that dream control is more likely when the lucidity is higher or more stable, and when the dreamer has more experience or practice with lucid dreaming (Mallett et al., 2021; Schredl et al., 2018; Stumbrys et al., 2014; Stumbrys & Erlacher, 2017). These factors could potentially influence the degree of thought variability and spontaneity. Future studies could investigate this possibility by collecting detailed information about the level of lucidity, control, and intentionality in each dream report. This approach could provide a fine-grained analysis of the role of cognitive control in shaping the flow of thought during sleep.
Our results not only offer theoretical value for understanding spontaneous thought, but also speak to the utility of using participants’ language patterns as an assessment of their thought dynamics. The use of language patterns has been gaining popularity in other fields but has rarely been applied to understand how thoughts arise and unfold over time. A notable exception is the use of the think-aloud paradigm (Raffaelli et al., 2021; Sripada & Taxali, 2020). Here we show that language patterns, assessed as either retrospective recall or during ecological momentary experience sampling, can be a marker of variability in our thought stream. Of note, such variability was sensitive to different levels of constraints and cognitive control, highlighting its usefulness in distinguishing disparate states. At the same time, this measure provides additional validity for the freely moving thought question presented in the wakeful mind wandering dataset. This self-report question was intended to capture the level of constraints placed on thought (Mills et al., 2021), and should theoretically be associated with higher levels of thought variability (i.e., increased semantic incoherence) as thoughts are more free to move from one to the next, or wander from topic to topic. Our results provide support for this prediction of more movement in thought, which has previously only been validated using other self-report measures (Mills et al., 2021) or measures derived from neural activity (Kam et al., 2021). The use of semantic incoherence as an objective and automated measure of thought variability in self-reports offers a tool that could be very useful in future work.
4.1 Limitations
One limitation of the current study is that the analyses did not account for demographic variability, psychiatric diagnoses, or affective state, all factors that are known to influence mind-wandering and other thought processes. Second, waking and dreaming thought are highly complex states, and it is likely that constructs other than cognitive control make unique contributions to the amount of thought variability. For example, the increased agency in lucid dreams might have reduced thought variability independently of insight. Similarly, other non-deliberate (i.e., automatic) constraints might have contributed to thought variability (Christoff et al., 2016). Third, semantic incoherence is only one of many approaches to measuring language and alternative measures might provide additional insights.
To investigate cognitive control in dreams, we utilized a large collection of public dream reports from an online forum. These dream reports are not necessarily representative of the large variety of conscious experiences that occur within sleep. It is likely that these dream reports are biased towards REM dreams, which are significantly more narrative and immersive than non-REM dreams (e.g., hypnagogic hallucinations). Investigating the thought variability present in the variety of non-REM dreams would be an interesting direction of future research, especially in the context of creativity.
We did not compare thought variability directly between waking and dreaming. The Dynamic Framework of Thought and other accounts make specific predictions about the relationship between waking and dreaming thought dynamics, but the large discrepancy between how our datasets were collected precluded deriving conclusive results from such an analysis. Dream reports in the current study were not collected immediately during or after they occurred, whereas waking reports were collected in real-time using experience sampling (Shiffman et al., 2008). Though dream reports are considered reliable accounts of experience (Windt, 2013), they are susceptible to memory fallacies (Rosen, 2013). Dream reports collected directly from REM awakenings differ substantially from morning reports, including a higher level of bizarreness (Kirberg, 2022). In this dataset, the longer gap between experience and reporting in the dream reports than the waking reports would have been a major confounding factor in any direct comparison between the two. Prior work using self-report measures of thought variability suggest that dreaming thought consists of similar levels of variability as task-independent waking thoughts, both of which show higher variability than task-dependent waking thoughts (Gross et al., 2021). Future work comparing waking and dreaming thoughts within-individuals using a serial-awakening paradigm (Siclari et al., 2013) and language measures would provide valuable additional insights into the mechanisms of thought generation.
4.2 Conclusion
The flow of thought is relentless. Mental contents are generated during almost all waking hours and reappear during sleep, but only a subset of these thoughts arise predictably and with conscious intent. Here, we show that thoughts are more predictable, at least content-wise, when supported by cognitive control, and that this principle is present during both waking and dreaming thought. Impairments in the ability to constrain thought variability and thought’s moment-to-moment movement may be one of the major factors that underlie clinically significant alterations in spontaneous thought. Furthermore, understanding the processes by which cognitive control reduces the erratic nature of thought might offer insights to support the development of future therapies.
Declarations
Resources
All datasets come from prior publications and information on their availability can be found in the original references. All analysis code is available in a public GitHub repository at https://github.com/remrama/thococo.
Acknowledgements
RM was supported by the National Institutes of Health under award number T32NS047987.
Disclosures
The authors declare no competing interests.